Latest Issue
Featured Articles
Workscapes: Bringing Art, Recovery, and Creativity to the SIOP Experience
The Society for Industrial and Organizational Psychology’s (SIOP) annual conference is a cornerstone event, traditionally…
Vicarious Trauma and the VOTE Index
Abstract: In this article, I reflect on my personal experience participating in the VOTE study…
Solo I-O: How Lone I-Os in Organizations Move the Needle
For much of the field’s history, I-O psychology has faced branding challenges (Nolan et al.,…
The Best Defense Is a Good Offense: How I-Os Can Proactively Defend I-O Values in Politics and Public Policy
Authors’ Note: Acknowledgements to Kayden Stockdale and Mike Zickar Industrial-organizational (I-O) psychologists have traditionally focused…
Using Executive Coaching to Enhance the Professional Development of Graduate Students
Abstract: Executive coaching is an individualized professional development program typically reserved for experienced business leaders.…
Trauma-Informed Tips for a Comfortable Conference Experience at the 2025 SIOP Annual Conference
The 2025 SIOP Annual Conference in Denver is a pinnacle event for many industrial-organizational (I-O)…
Search TIP Articles
Article Type
Topic
Issue
IOtas
IOtas celebrates SIOP member achievements like awards, job changes, and publications. Fill out the form…
Members in the Media
Awareness of I-O psychology has been on the rise thanks to articles written by and/or…
I-O Education Gaps and Potential Solutions: A Town Hall Discussion at SIOP 2025
Author Note: We have no known conflicts of interest to disclose. This material was presented at…
The 2025 SIOP Conference
Note. The views expressed in this article are those of the authors and do not…
Workscapes: Bringing Art, Recovery, and Creativity to the SIOP Experience
The Society for Industrial and Organizational Psychology’s (SIOP) annual conference is a cornerstone event, traditionally…
Vicarious Trauma and the VOTE Index
Abstract: In this article, I reflect on my personal experience participating in the VOTE study…
Issue Archive
Access more than 50 years of I-O news. From the first Division 14 newsletter in 1964, all back issues are available in PDF format.
Other Useful Links
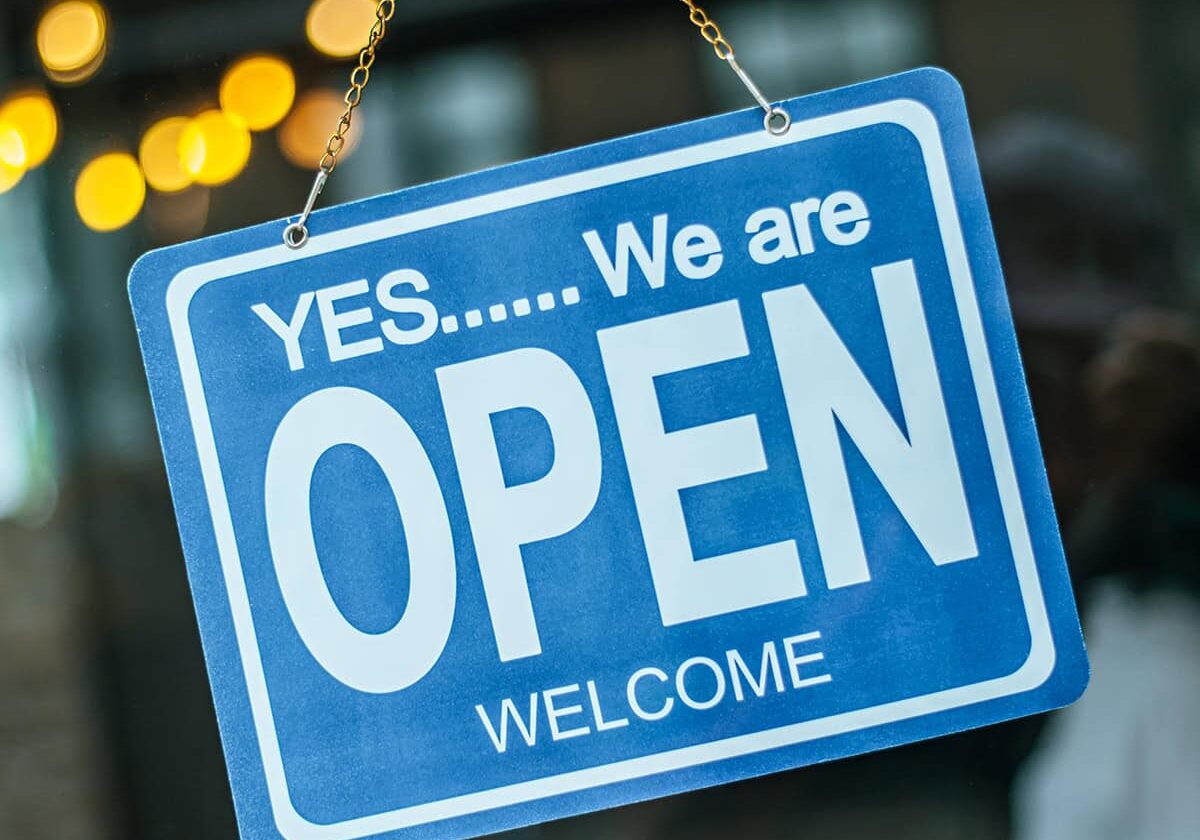
Advertising
Put your ad in front of thousands of I-O psychologists.
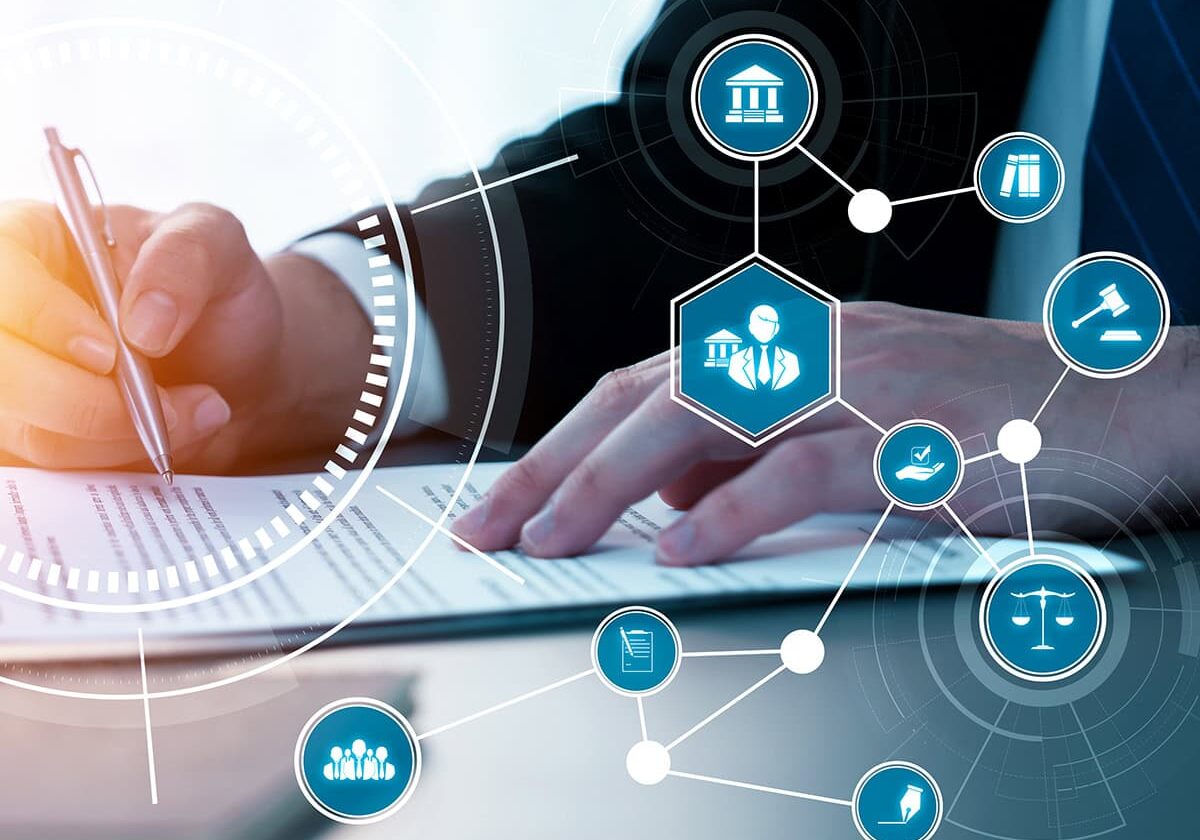
TIP Policies
Get published! Information about submission types, word limits, and deadlines available here.
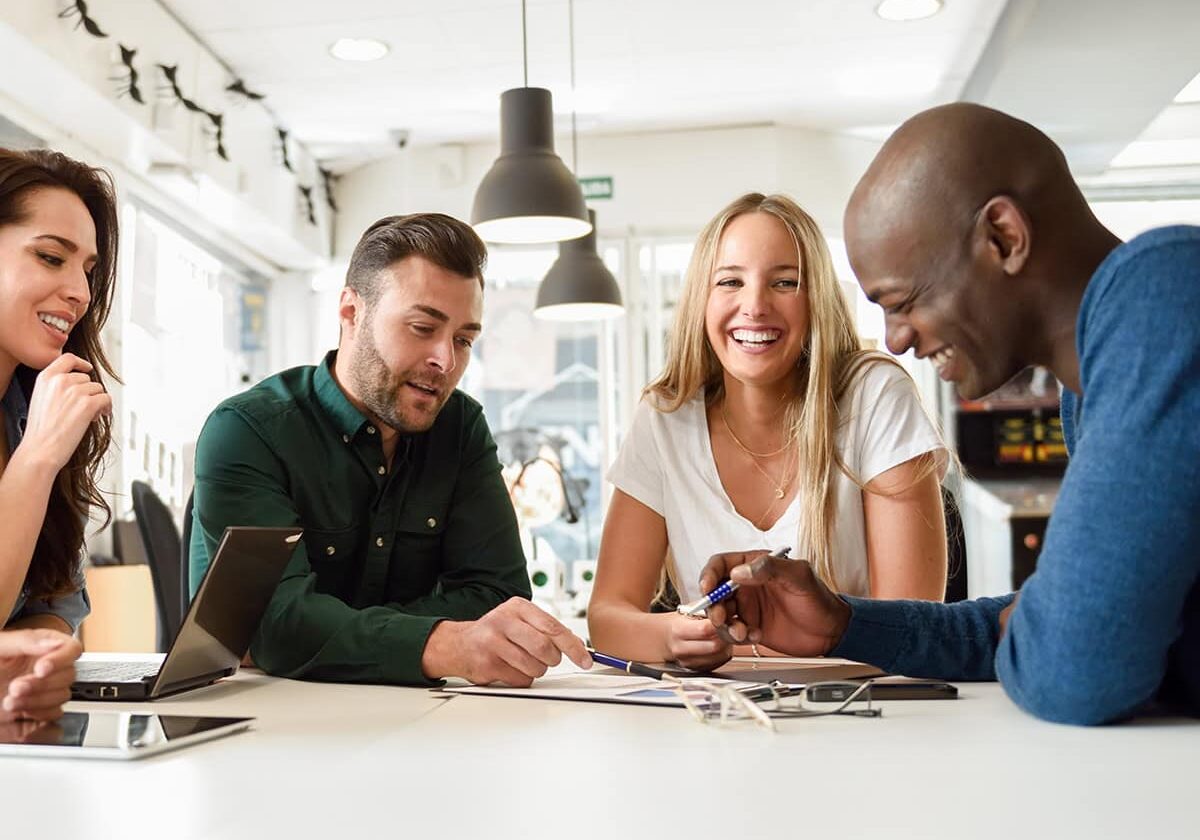
Board
The TIP editor and board strive to provide the best and most relevant information to SIOP members and other TIP readers.
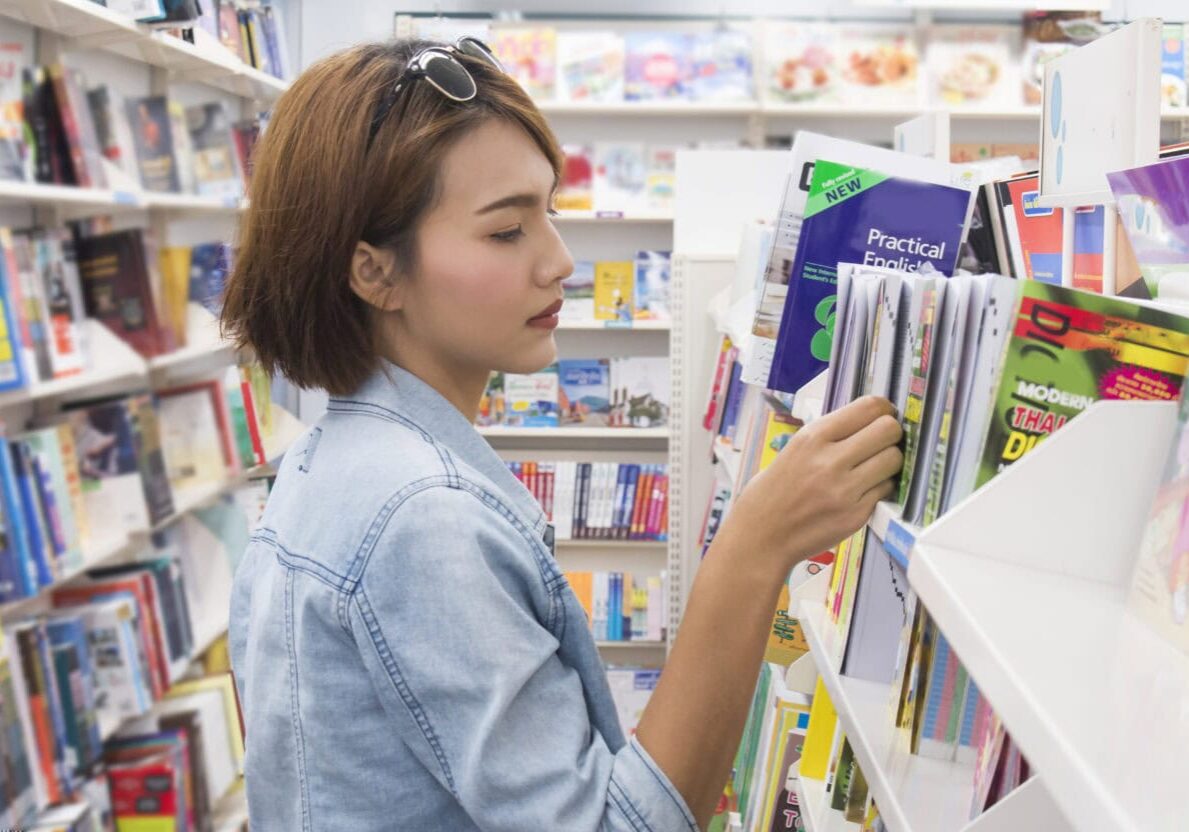
Submit to IOtas
IOtas is a TIP column designed to announce accomplishments of SIOP members. Send your news or brag about a colleague, and please include a photo!