Latest Issue
Featured Articles
Protected: Workscapes: Bringing Art, Recovery, and Creativity to the SIOP Experience
There is no excerpt because this is a protected post.
Protected: Vicarious Trauma and the VOTE Index
There is no excerpt because this is a protected post.
Protected: Solo I-O: How Lone I-Os in Organizations Move the Needle
There is no excerpt because this is a protected post.
Protected: The Best Defense Is a Good Offense: How I-Os Can Proactively Defend I-O Values in Politics and Public Policy
There is no excerpt because this is a protected post.
Using Executive Coaching to Enhance the Professional Development of Graduate Students
Abstract: Executive coaching is an individualized professional development program typically reserved for experienced business leaders.…
Trauma-Informed Tips for a Comfortable Conference Experience at the 2025 SIOP Annual Conference
The 2025 SIOP Annual Conference in Denver is a pinnacle event for many industrial-organizational (I-O)…
Search TIP Articles
Article Type
Topic
Issue
Protected: I-O Education Gaps and Potential Solutions: A Town Hall Discussion at SIOP 2025
There is no excerpt because this is a protected post.
Protected: Workscapes: Bringing Art, Recovery, and Creativity to the SIOP Experience
There is no excerpt because this is a protected post.
Protected: Vicarious Trauma and the VOTE Index
There is no excerpt because this is a protected post.
Protected: Solo I-O: How Lone I-Os in Organizations Move the Needle
There is no excerpt because this is a protected post.
Protected: The Best Defense Is a Good Offense: How I-Os Can Proactively Defend I-O Values in Politics and Public Policy
There is no excerpt because this is a protected post.
Issue Archive
Access more than 50 years of I-O news. From the first Division 14 newsletter in 1964, all back issues are available in PDF format.
Other Useful Links
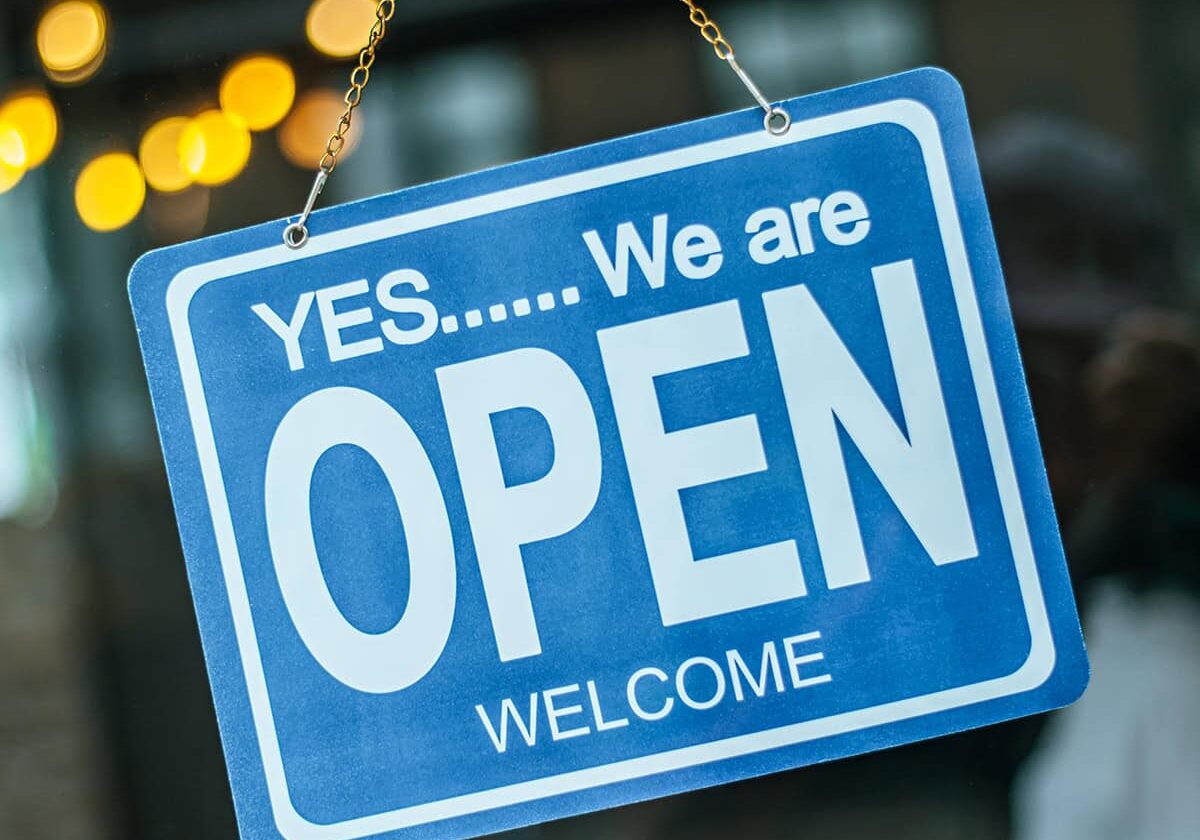
Advertising
Put your ad in front of thousands of I-O psychologists.
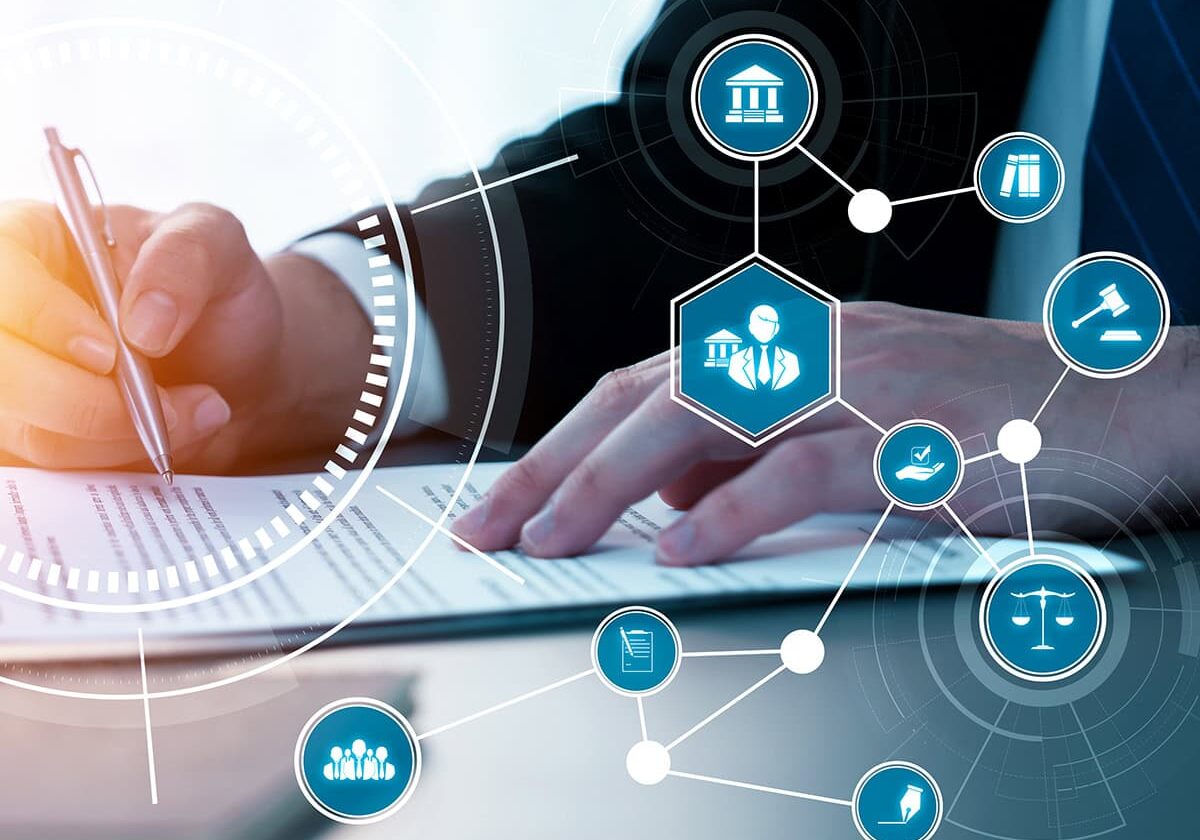
TIP Policies
Get published! Information about submission types, word limits, and deadlines available here.
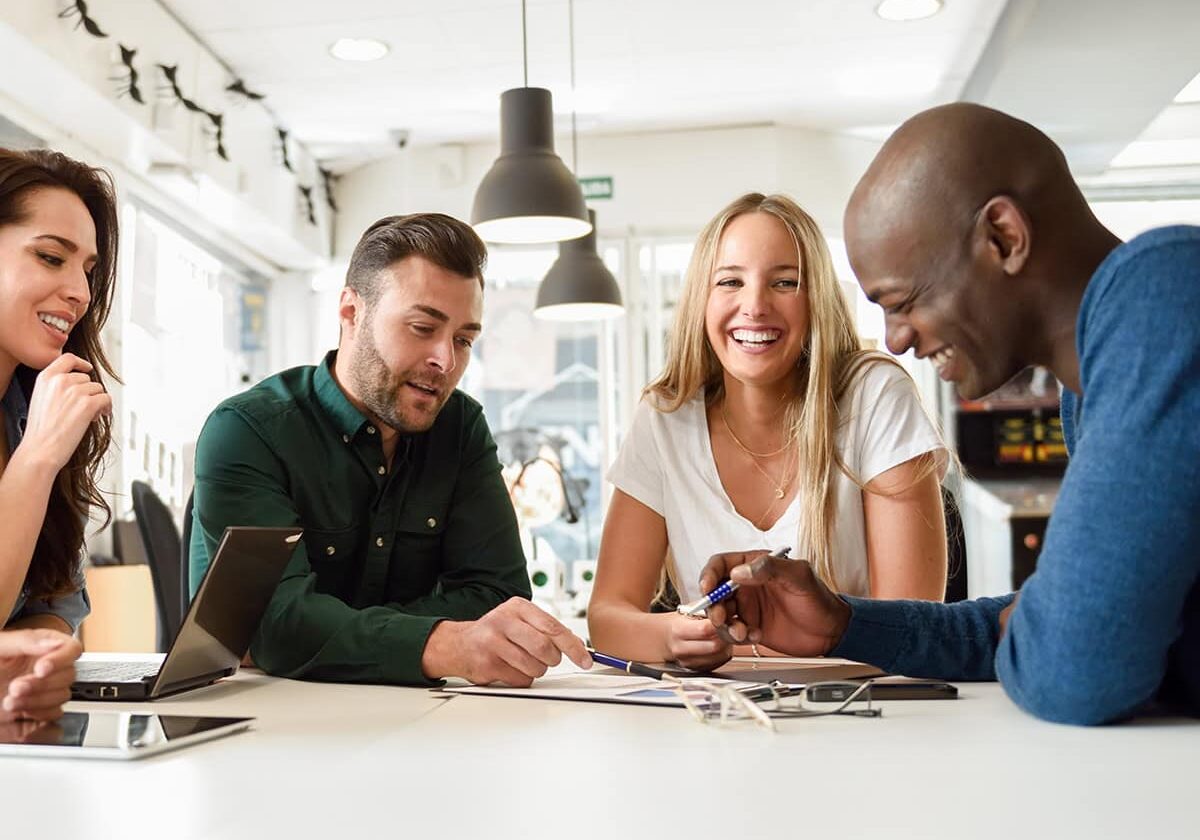
Board
The TIP editor and board strive to provide the best and most relevant information to SIOP members and other TIP readers.
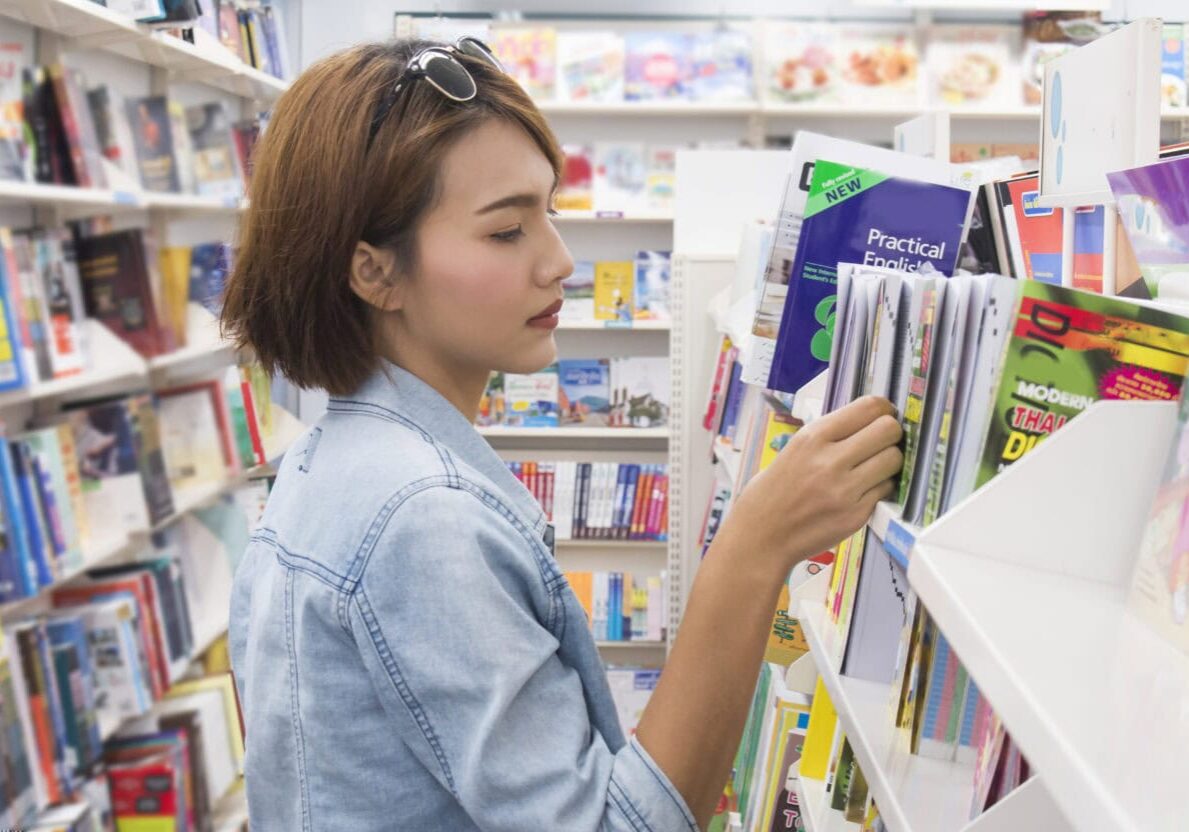
Submit to IOtas
IOtas is a TIP column designed to announce accomplishments of SIOP members. Send your news or brag about a colleague, and please include a photo!